The community and network post I started with this week seems to have gotten me into a vocabulary mood. Here I'll share some thoughts on "qualitative" and "quantitative" research.
Or one thought: it's a silly distinction.
About 2500 years ago, Plato and Aristotle proposed 2 ways of conceptualizing the world. Plato focused on abstractions and ideals while Aristotle focused on empirical and practical issues.
Aristotelian thought has been valuable for what we call natural sciences. Classification is the king of methodologies in this thought tradition, and biology has been a major beneficiary. This mode of thought is associated with the rationalism and technology gains of the European medieval and renaissance era.

https://www.pinterest.com/pin/493566440386147691/
Platonic thought is valuable for what we call the hard sciences, where math can clearly describe what we observe in the world. After Newton (and others) created calculus to describe motion around the beginning of the 18th century, this mode of thought had a revival and dominated European philosophy through the industrial revolution.
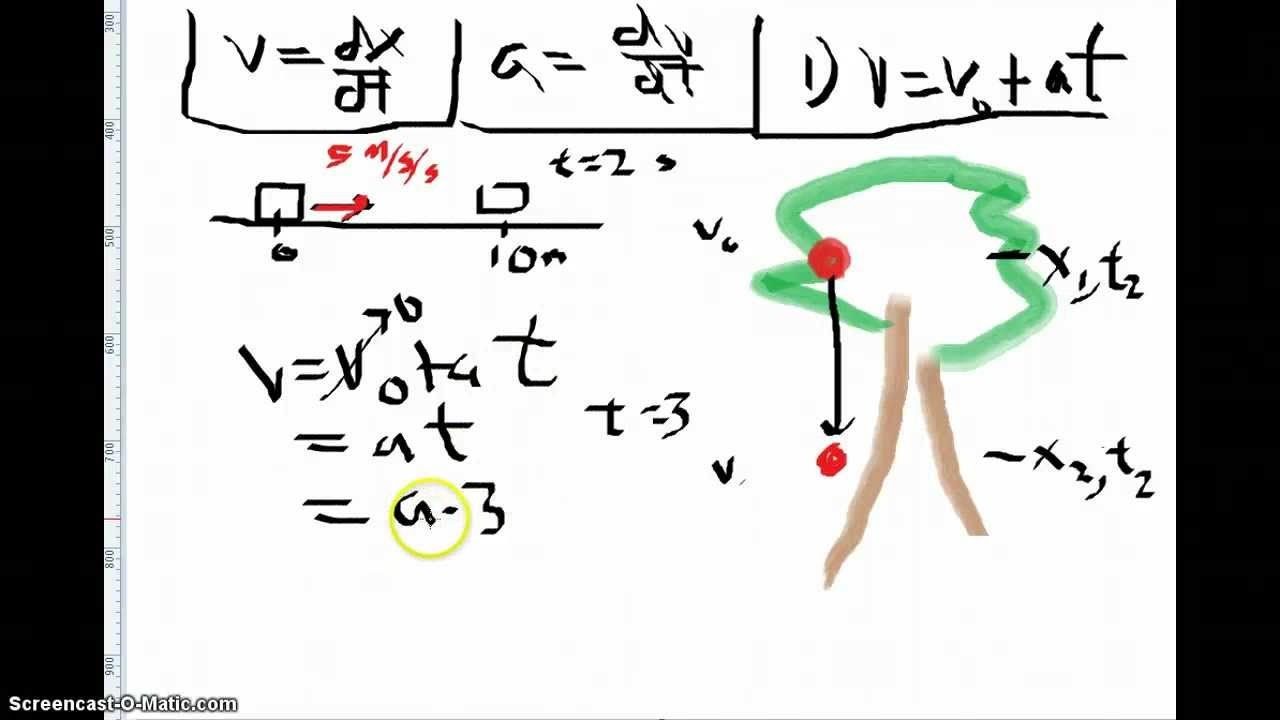
https://www.youtube.com/watch?v=ShiWQ5vqEkY
But then, about 100 years ago, Einstein and his contemporaries ran into the limitations of this method; they could not adequately describe the world in abstract terms. Twentieth-century scientists from Whitehead to Hawking pointed out the need to draw on all available powers of observation, abstraction, and logic to compensate for the limitations of each individual method and improve our collective understanding.
In fields like environmental science, where all viable methods are welcomed for their ability to contribute knowledge about a phenomenon, we have made good progress. Our success with the hole in the ozone layer during the late 20th century is a testament to the power of modern holistic thinking.
For a fascinating look at this history up to the early 20th century, explore
Whitehead, A.N. 1929. Science and the Modern World: Lowell Lectures 1925. Cambridge University Press, London.